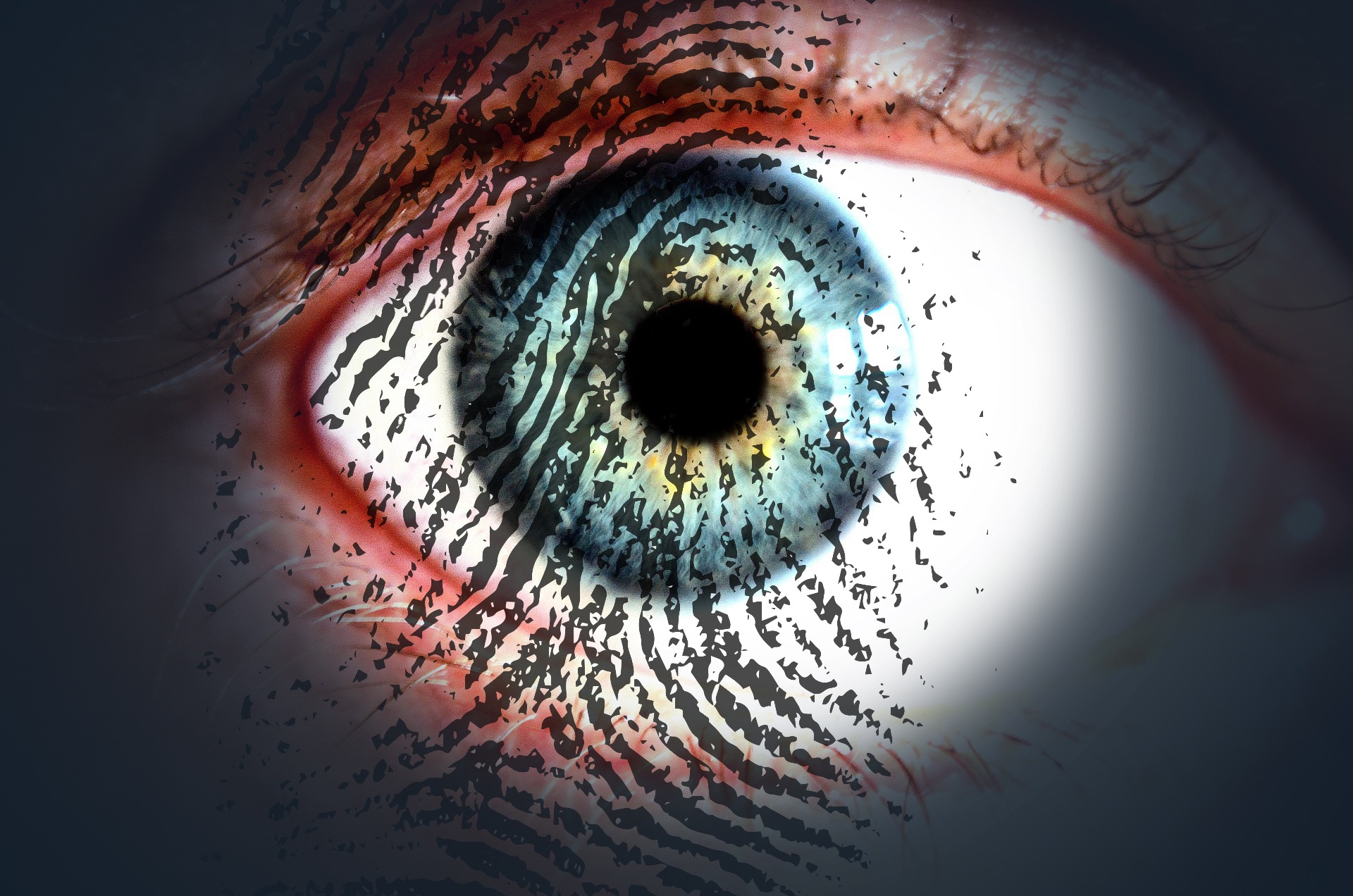
Another V:
Making The Case for Big Data Veracity
In a presentation made at the San Diego joint NIST/ JTC1 Big Data meeting in March 2014, I argued for Provenance as a major concern of Big Data standards organization. I am proposing Veracity as the fourth V in the Big Data V’s, and suggest that veracity is a useful near-synonym for provenance. Whether veracity/provenance is a subset of metadata, or vice versa, is a fruitful discussion worth having. In the meantime, these seven reasons are recommended as part of the rationale for the inclusion:
- In the absence of provenance information, data can be incorrect while fully secured.
- Privacy concerns continue to be the primary interest of the public in Big Data. The legacy of big data providers in credit scoring (Experian, TransUnion, etc.) has led to mistrust in the ability of individuals to own, and to correct data about themselves. This concern is not addressed in the other V’s. It is a problem not addressed by simply securing incorrect data. Without making veracity a top level concern, the Big Data standards activity is likely to be perceived as self-serving geek-speak that ignores these legitimate concerns.
- Because re-identification, complex event processing, digital forensics and data fusion are activities that already ingest Big Data, the potential for misattribution, error and faulty inference-making will increase.
- The Internet of Things will add a huge overlay of machine-to-machine data for which automated systems will rely. Establishing provenance for each individual sensor – especially firmware/software-based sensors – is domain-specific; for instance, it could require nontrivial calibration that is configuration-aware and time-critical. In addition, such provenance data is potentially a Big Data source itself.
- Big Data systems must be designed with user interfaces that take into account human factors aspects of sensor data. For instance, some sensors may be critical in whose absence automated systems should be halted. In other settings, such as dashboards, different sensors can be substituted. The process of degrading a Big Data system that requires on high volume, high velocity data streams could well entail intelligent use of specific metadata and provenance elements.
- Building resilient systems, or understanding the feasibility of an approach to resilience, requires an integrated approach to data source quality, reliability. Big Data systems that rely on aggregate data must take into account any limitations of those sources. Data availability and timeliness (e.g., information on demand), for instance, may be a feature of provenance. For example, some data sources may be available only at scheduled times, or only when dependent systems are healthy, or even upon power or weather conditions.
- GRC issues may apply that are not captured elsewhere. For instance, data could be rented and cannot be used after the rental period. Royalty payments may be derived based upon provenance data elements. Data may be legally licensed for use only in certain countries, or only in limited contexts. Data could be subject to court ordered retention and formatted regulations. The other V’s do not capture any of this.
Refer to the widely distributed IBM infographic. An IBM-sponsored study Big Data found that lack of confidence in data sources is a major concern. As summarized by the Wall Street Journal.
A fragmented approach can result in a breakdown of trust among different groups of people who may be accessing, interpreting, and using data in different ways. This gap stems from a basic distrust about who is qualified to competently analyze and act upon the data. A lack of trust among executives, analysts and data managers can significantly impact the willingness to share data, rely on insights and work together to deliver value. IBM’s study found that a trust gap among individuals is a leading indicator of lack of trust in the veracity of data. When this happens, the overall costs to a business are high.
Other Sources Recommending Big Data V=Veracity
Inside Big Data http://inside-bigdata.com/2013/09/12/beyond-volume-variety-velocity-issue-big-data-veracity/
Patricia Saporito (SAP blogger) http://blogs.sap.com/innovation/big-data/2-more-big-data-vs-value-and-veracity-01242817
Objectivity.com http://www.objectivity.com/news/blog/gartners-missing-vs-value-and-veracity/#.U1
Big Data for Dummies Cheat Sheet http://www.dummies.com/how-to/content/defining-big-data-volume-velocity-and-variety.html